Unlocking insights from unstructured clinical data with generative AI
By Light-it, in collaboration with Annie Chiang, Clinical AI Data Scientist.
Technology adoption in healthcare is traditionally cautious and slow, however, just a few years after ChatGPT burst onto the scene, ambient AI scribes have been deployed system-wide at major healthcare organizations and parallel efforts are underway to automate many healthcare administrative tasks. Drawing from years of working with unstructured oncology data, I am excited by the treasure trove hidden in unstructured clinical data that can be unlocked with Generative AI (GenAI) technologies. Specifically, GenAI has the potential to improve patient and provider experiences significantly. Here, I will highlight a few promising efforts of applying GenAI to unstructured clinical data, which account for >80% of all clinical data, as well as an evaluation-based framework needed for production deployment of such GenAI powered tools.
GenAI enabled patient engagement
Patient engagement is generally defined as the active participation of a patient in their own health, treatment and management. Hitherto, patient engagement has been low, and experts believe it contributes to suboptimal health outcomes. Oftentimes, patient engagement is hampered by lack of personalized health education, lack of timely provider communications and/or other system-related problems. Today, ongoing pilots are testing GenAI technologies to create and tailor patient educational materials so that it can be easier to understand (e.g. translating complicated medical jargon into lay language) in order to better manage their health, including Ana, a GenAI search tool developed by Google in partnership with American Cancer Society to help cancer patients navigate through their cancer journey. Moreover, GenAI can generate the same materials to be consumed in different modes. Thus, the same content can be consumed via an interactive chatbot interface using text or voice – which is preferred by older adults, or through multimedia interface.
Another benefit from healthcare GenAI tools is in empowering patients. Spurred by the 21st Century Cures Act, patients are able to aggregate their medical records from various providers, which allows them to share their medical context with their care team from their laptop. In effect, it may facilitate easier ‘second opinions’ based on more comprehensive medical history. This level of portability of personal medical information on a population scale can at once reduce redundant costs (e.g. repeat lab tests) and improve the patient experience. Fundamentally, this shift empowers the patient to take charge of their health journey.
GenAI enabled clinical decision support
The rapid pace of biomedical progress has increased the burden of providing evidence-based, standard of care, such that even specialists can struggle to keep up to date with the latest advances. This is especially true in oncology, where personalized treatment plans require synthesis of different data sources (e.g. prior medical history from many providers, biomarker status from genomic tests), established clinical guidelines and emerging evidence found in published literature. This time-consuming workflow led to the development of Capricorn, an AI Clinical Decision Support (CDS) tool built by Google using Gemini models, in collaboration with Princess Máxima Center for pediatric oncology in the Netherlands. Capricorn is able to shorten workflows required to synthesize past medical history, primary literature, and relevant de-identified data from days to minutes in order to provide personalized treatment plans for regular tumor board discussions.
Another GenAI-powered CDS is Color Health’s Cancer Copilot. It converts established National Comprehensive Cancer Network (NCCN) guidelines into knowledge bases that generate personalized lists of diagnostic workups for newly diagnosed cancer patients, saving valuable time. To be clear, both Capricorn and Color’s Cancer Copilot are reducing the burden of providing personalized care, yet allowing for clinical nuance and judgment.
Evaluation-driven GenAI powered product development
It has never been easier to develop POC or vibe code an app, however, to build healthcare GenAI powered tools requires a thoughtful framework that places data quality and evaluations at the center (see figure).
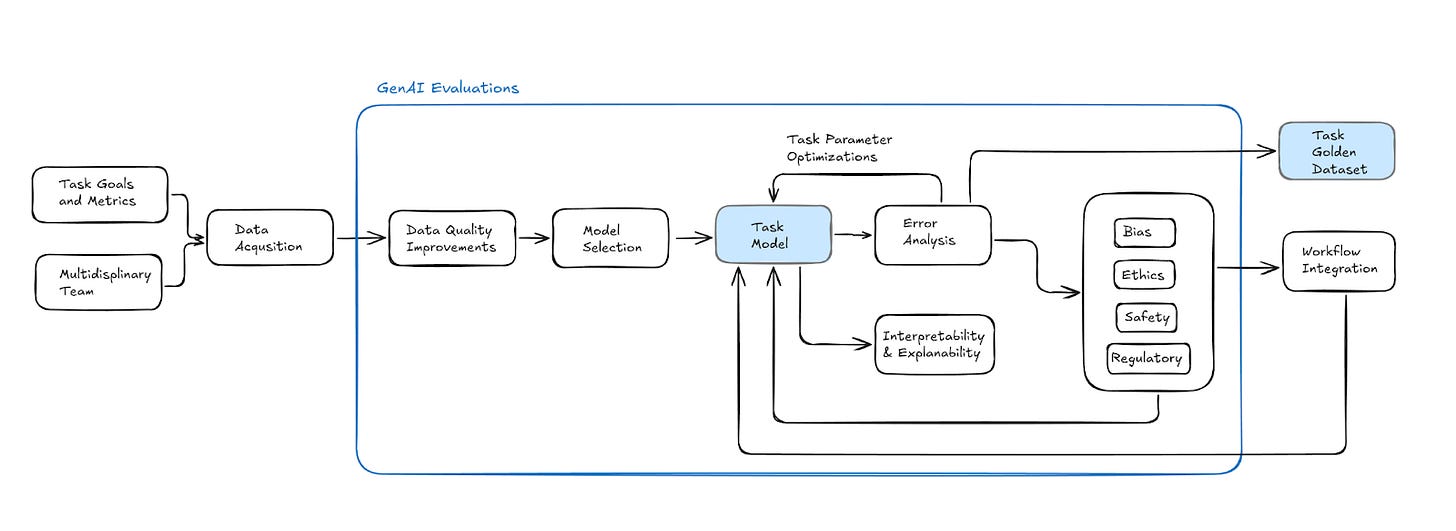
To deploy GenAI applications in healthcare, domain experts should co-lead the product development by helping to define goals and highlight blind spots. Let’s walk through an example of a patient's medical record summarization from their clinical notes. First, separating clinical notes by type, in other words, separating the processing of imaging results from primary visit notes, allows the models to specialize and increase performance. This may apply to other types of notes such as lab test results and medication. Possible metrics include the accurate identification of relevant clinical terms (named entity recognition) and dates. Next, improving data quality such as resolving missing data and duplications, standardizing data (e.g. SNOMED-CT, RxNorm) and capturing of causal relationships (e.g. pembrolizumab for non-small cell lung cancer) are crucial to downstream uses.
Once the data is processed and standardized (resulting in higher-quality data), comparing the results of a small but representative test dataset should yield an optimal model, given performance and cost considerations. General models such as ChatGPT or Claude may not yield high performance in clinical applications, thus, rigorous error analysis and parameter optimizations are needed. Wherever possible, the interpretability and explainability of the decision-making process will help in gaining trust of users.
Once the test set evaluations are sufficient to produce a golden dataset, additional assessments of bias, ethics, safety and regulatory compliance is needed before the most important step in the entire framework - workflow integration. While captured as the final step, domain experts should be part of the multidisciplinary team and clinical workflow considerations included as part of the product specification. Only then, will the GenAI product be successfully integrated into care delivery. Perhaps more than the development of other applications, the GenAI-powered apps require continuous monitoring. This is reflected in the feedback loops back to the model to adjust various parameters. While the framework may seem daunting, it is vital to gain trust and reach wide adoption.
GenAI can transform healthcare at scale
Proportional to all data generated globally, healthcare data currently make up roughly 30%, with unstructured data being the primary driver. Deriving insights from large datasets creates tremendous burden. Thankfully, one of the superpowers of GenAI technologies is that they are able to separate signals from noise. As shown in the figure, the development of GenAI powered clinical tools is not for the faint of heart, given that it takes a long time horizon, considerable resources, and alignment. However, the early prototypes of such GenAI applications applied to patient engagement and clinical decision support have shown that it has the potential to transform the healthcare industry at scale. I am hopeful that these applications are just the tip of the iceberg and that we can move closer to having the right care at the right time for all.
Thanks for reading DHI’s HealthTech Expert Column!
We hope you’ve learned as much as we did with this piece.
Stay tuned for next month’s Expert Column to keep gaining insights from various experts and stay ahead of the curve in digital health innovation!
Want to join the conversation? We’re always looking for new guest columnists to share their expertise with our audience.
Reach out if you’d like to be featured!